Investigating neutrino collective flavor oscillations with machine learning
During the explosion of a massive dying star — a supernova — millions of tiny subatomic particles called neutrinos are emitted. These neutrinos are emitted from deep inside the star, and can diffuse through the stellar envelope even when photons are trapped, and hence, act as the first messengers of the explosion. most of the gravitational binding energy of the star is through these tiny particles, and therefore, the study of their travel from the distant star to earth detectors is of primary atrophysical interest.
Neutrinos are found in three flavours in nature, electron, muon and tau. Once emitted, their flavors oscillate, and this flavor evolution is impacted by both the surrounding stellar medium and interactions among themselves. As the electron is the one most easily detected, a lot of effort has been devoted to understanding how flavor evolves and how earth detectors can be used to infer various properties of the explosion.
Currently, the evolution of neutrino flavor in dense environments such as core-collapse supernovae and binary compact object mergers constitutes an important and unsolved problem. Its solution has potential implications for the dynamics and heavy-element nucleosynthesis in these environments.
We combine data assimilation, ordinary differential equation solvers, and deep neural networks to craft an inference approach tailored for non-linear dynamical systems. Using this architecture, and a simple two-neutrino-beam, two-flavor model, we compare the performances of nine different optimization algorithms and expand upon previous assessments of the efficacy of inference for tackling problems in flavor evolution.
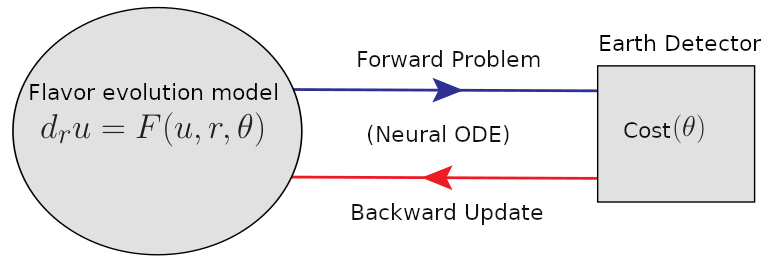
We find that employing this new architecture, together with evolutionary optimization algorithms, accurately captures flavor histories in the small-scale model and allows us to quickly explore both model parameters and initial flavor content. This study shows great promise for the application of machine learning in the study of complicated astrophysical phenomena such as neutrino collective flavor oscillations.
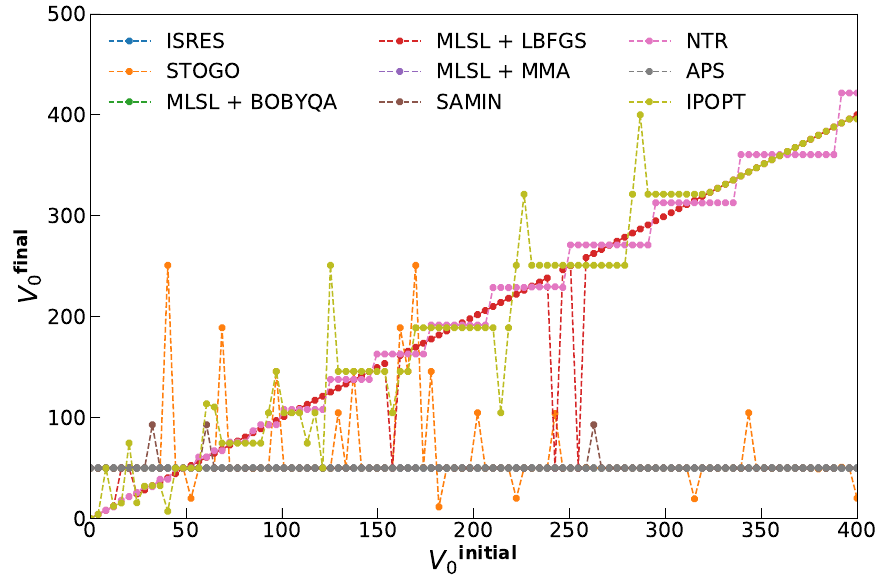
This introduction was prepared by N3AS fellow Ermal Rrapaj. Credit for header graphic supernova: MPIA/NASA/Calar Alto Observatory.
Read More: